GrowthPhysics is a data-driven growth agency that leverages financial modeling, engineering, and marketing to deliver outlier growth for clients. Our proprietary financial models and seasoned full-stack growth team analyze and identify the right growth program for your current growth stage to execute and optimize the entire funnel.Â
Read on for their guest contribution to the Iterable blog to learn how their RFM methodology can change the way you look at customer lifetime value.Â
The goal of every consumer brand is to maximize customer lifetime value (LTV). Short-term relationships with customers end up costly. Brands are better off building long-lasting relationships that benefit the organization and the customer.
Part of this process means identifying which customers are the most valuable to retain. In our retention practice at GrowthPhysics, we recommend a Recency Frequency Monetary (RFM) framework that is the secret centerpiece to optimize stronger customer relationships.
Below we outline exactly what the RFM methodology entails and an easy 5-step implementation process to show how you can use data-driven customer segmentation to increase your LTV.
The Power of RFM for Customer Lifetime Value
Simply put, RFM is a dynamic customer scoring methodology for assigning each customer a value based on historical purchase data. For example, direct-to-consumer (DTC) brands’ clearest signal of value is a purchase.
However, the RFM methodology uses three behavior attributes of a purchase to dig deeper into a customer’s value to a brand:
- R = Recency of purchase
- F = Frequency of purchase
- M = Monetary value of purchase
When it comes to customer lifetime value, RFM enables brands to understand their customers’ activities at a more granular, segmented level. In turn, the messaging and promotions can be tailored to the determined value in the RFM methodology. This enhanced visibility into behavioral data gives brands actionable insights.
In practice, RFM looks a bit like this:
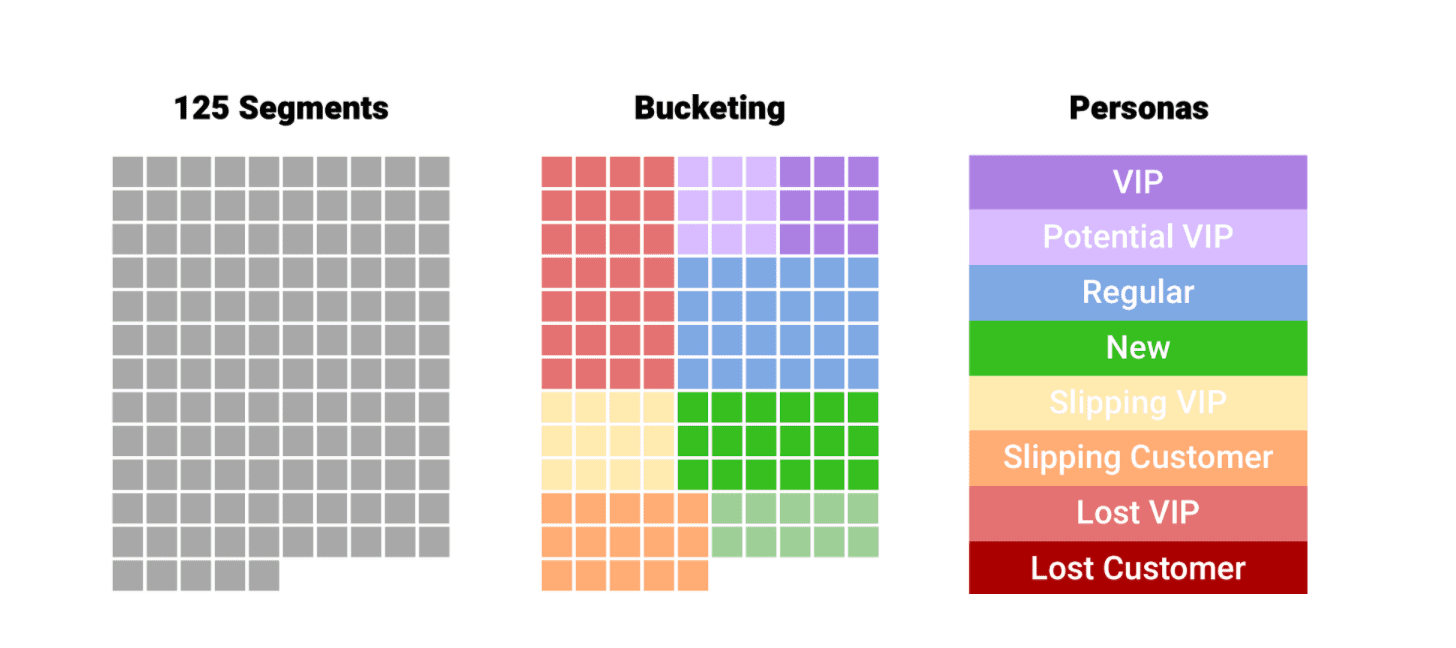
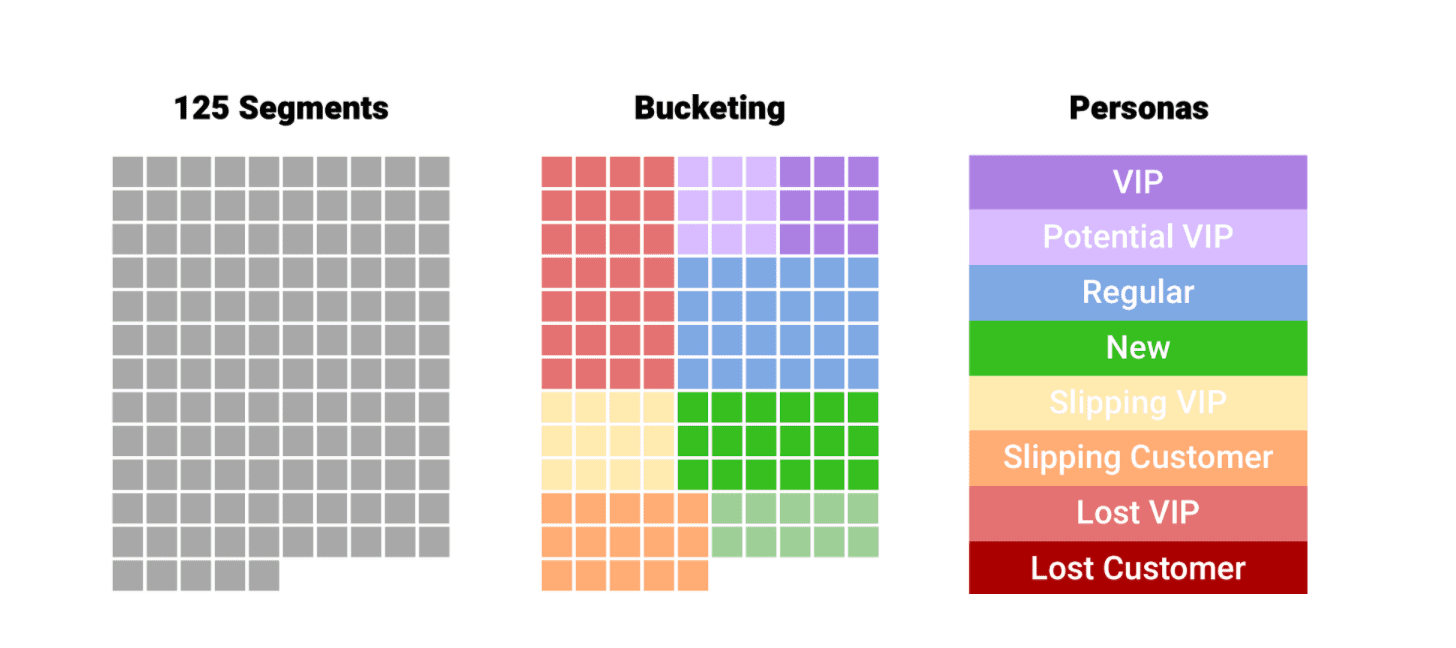
Example of how RFM can segment customers into personas.
A typical RFM implementation assigns a value of 1 to 5 for each of the Recency, Frequency, and Monetary attributes. The 5x5x5 matrix results in 125 customer segments you see here on the left. Based on the assigned values, customers are further segmented into buckets.
In our practice, we bucket the segments into a value spectrum of 8 personas based on variations of 3 customer types: VIP, Regular, and New Customer. The gradations of customer type allow you to personalize content and messaging by bucket in a way that more closely aligns with the customer’s behavior.
Customer lifetime value insights at this level make it exponentially easier to quickly spot positive or negative movement along the RFM spectrum to strengthen relationships and mitigate churn.
Exciting, right? Here’s how easy it is to execute with GrowthPhysics and Iterable.
A 5-Step Process For Maximizing Retention
Our overarching objective in retention engagements is to maximize your customer lifetime value.
To get there, this 5-step implementation process combines the power of RFM with Iterable’s cross-channel capabilities on top of a Shopify, Webflow, or custom stack to make the most of your relationships with customers.
Step 1: Install RFM Framework
The heavy lifting is to implement an RFM machine learning model to segment customers. There are plenty of DIY blogs and whitepapers on this subject matter using Python and pandas.
The goal is to enrich your Iterable instance with RFM artifacts, segments and personas, so marketers can create messaging strategies based on RFM targeting. Here is how we do it.
We make RFM implementation easy. If you are on Shopify, simply install our GrowthPhysics Shopify app and instantly have RFM implemented on your store.
We enrich the customer table with an rfm object with two properties:
rfm.score
rfm.persona
We use rfm.persona
inside Iterable for value-driven message targeting. While we inject rfm.score
into UTMs for downstream segmentation analysis inside analytics platforms (see step 4).
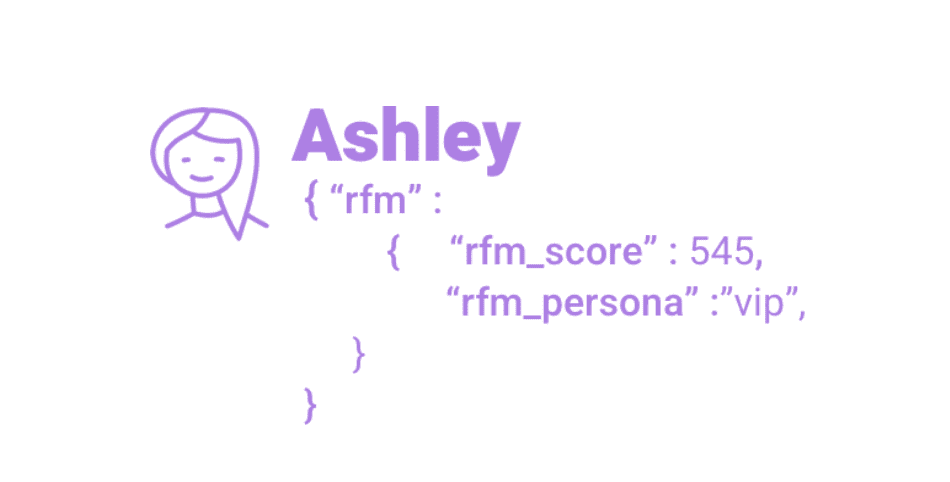
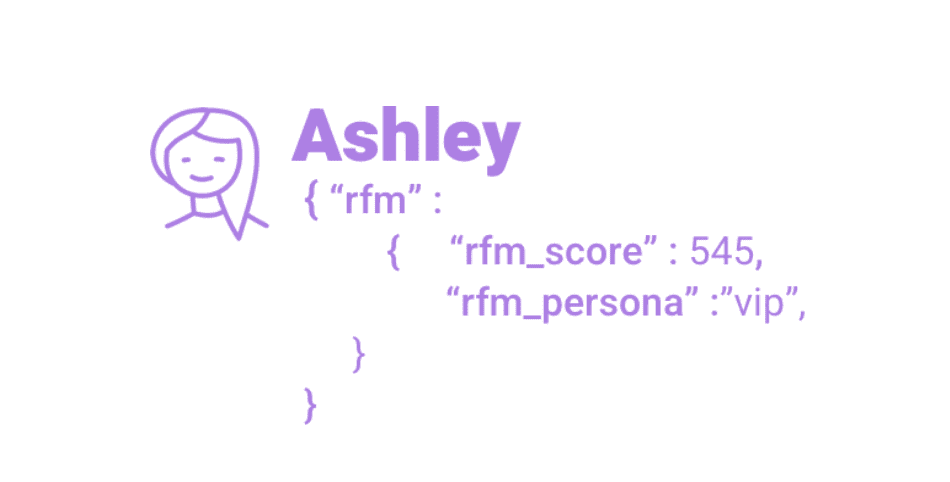
The RFM score and persona are added to the customer table.
Step 2: Inject Data Personalization Attributes
Just RFM alone does not enable a highly personalized and relevant messaging strategy. Attributes used directly via handlebar injection or indirectly via handlebar logic are ideal for personalization within an RFM framework. One such attribute for DTC brands is a customer’s favorite product or favorite category based on purchase behavior.
Our app augments the customer table with a favorites object.
favorites.product.*
favorites.category.*
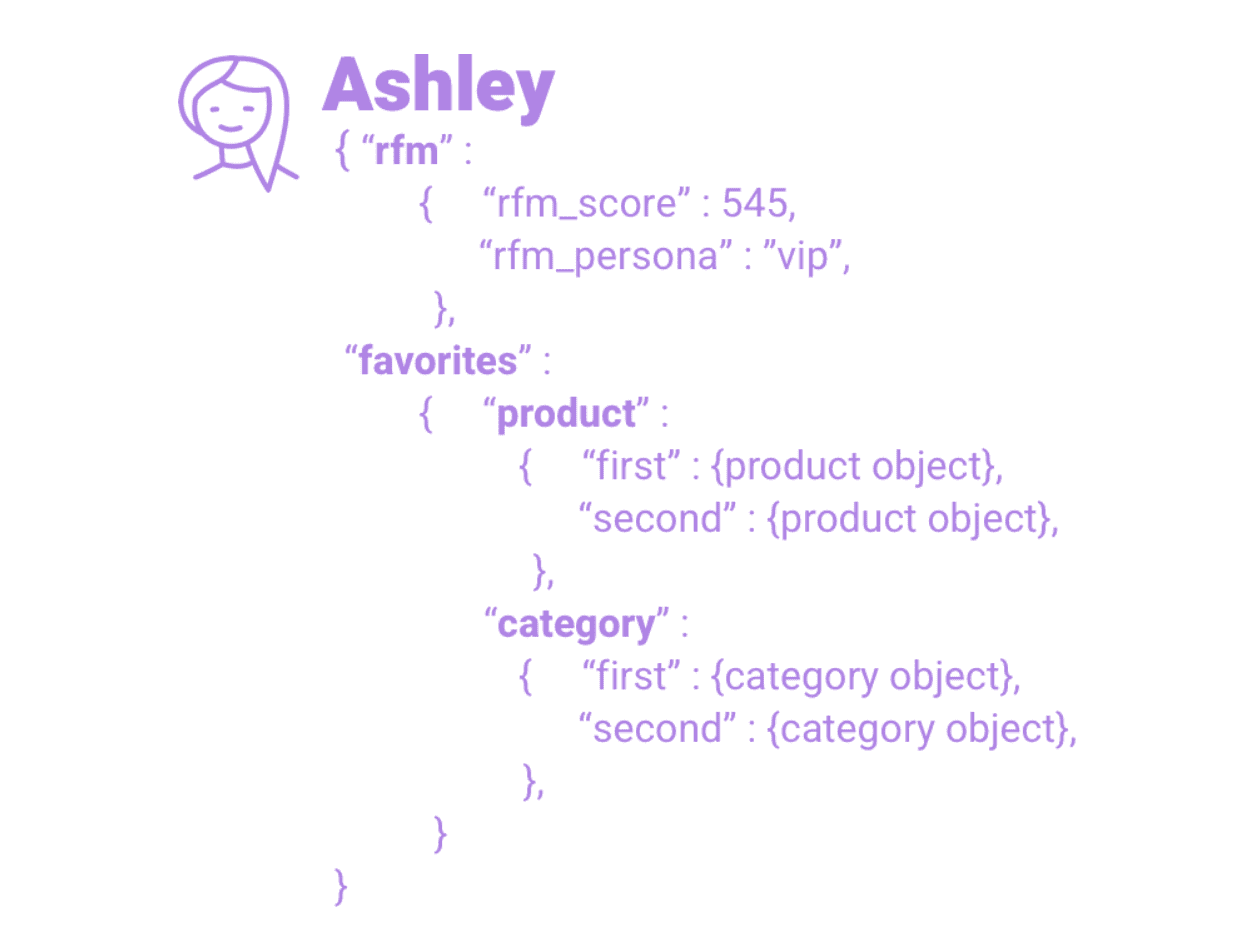
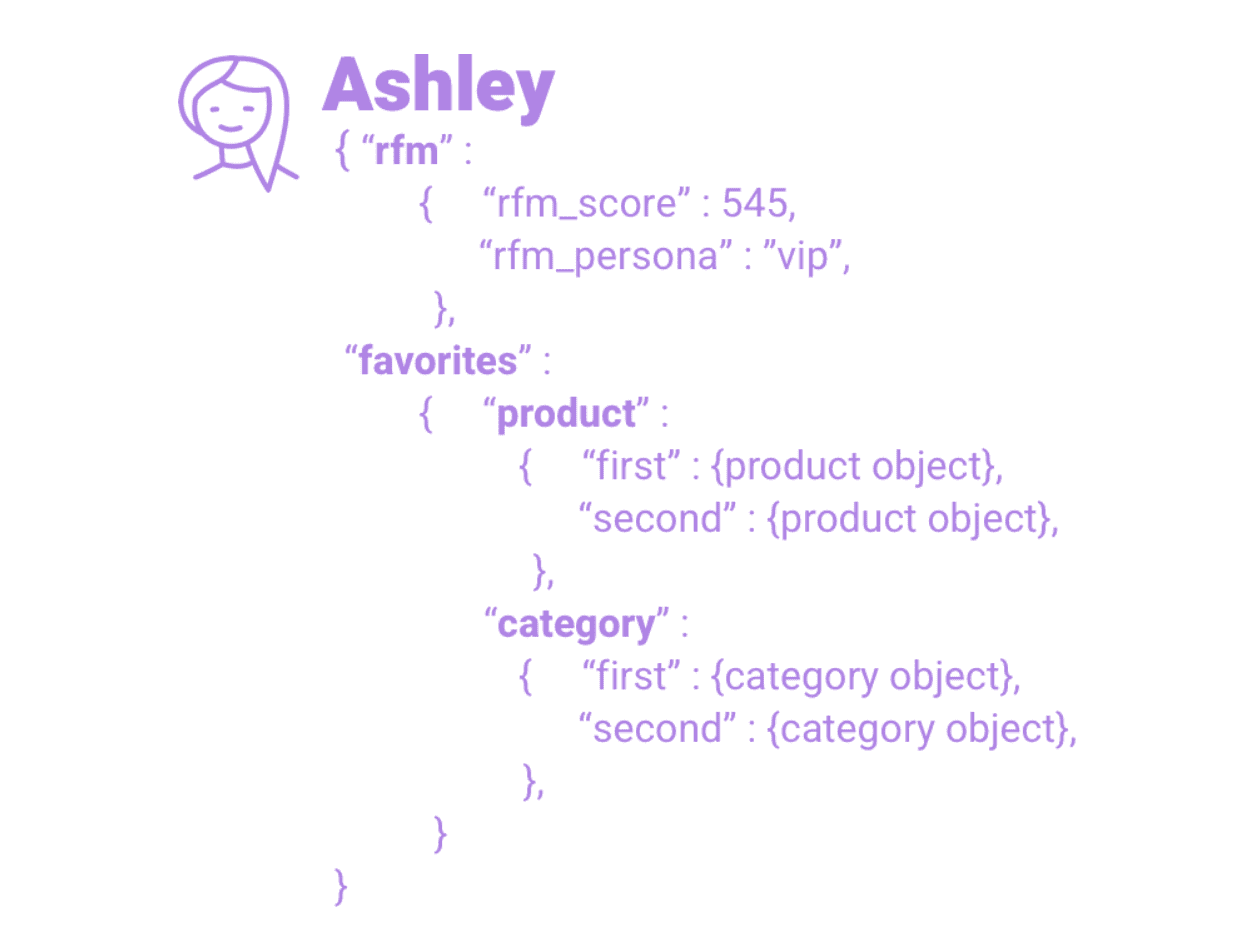
To enhance personalization, “product” and “category” can be added to the customer table as well.
From here, you can directly inject a customer’s favorite product into the messaging. You can even recommend a new SKU based on the customer’s favorite category or other products based on a favorite product’s or favorite category’s purchase correlation.
Step 3: Catalog and Data Feeds
Product catalogs and relevant data feeds, such as weather, provide additional information not available in the Customer Properties. These data feeds can add further personalization elements or relevant context into the messaging experience.
For example, a weather context can be used to show different products within a favorite category.
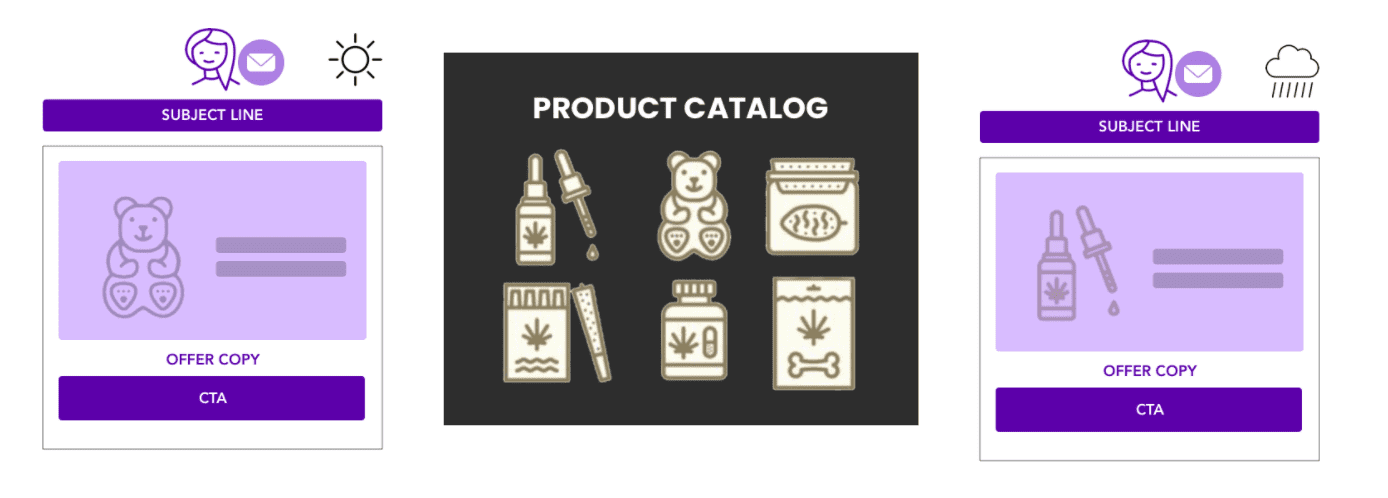
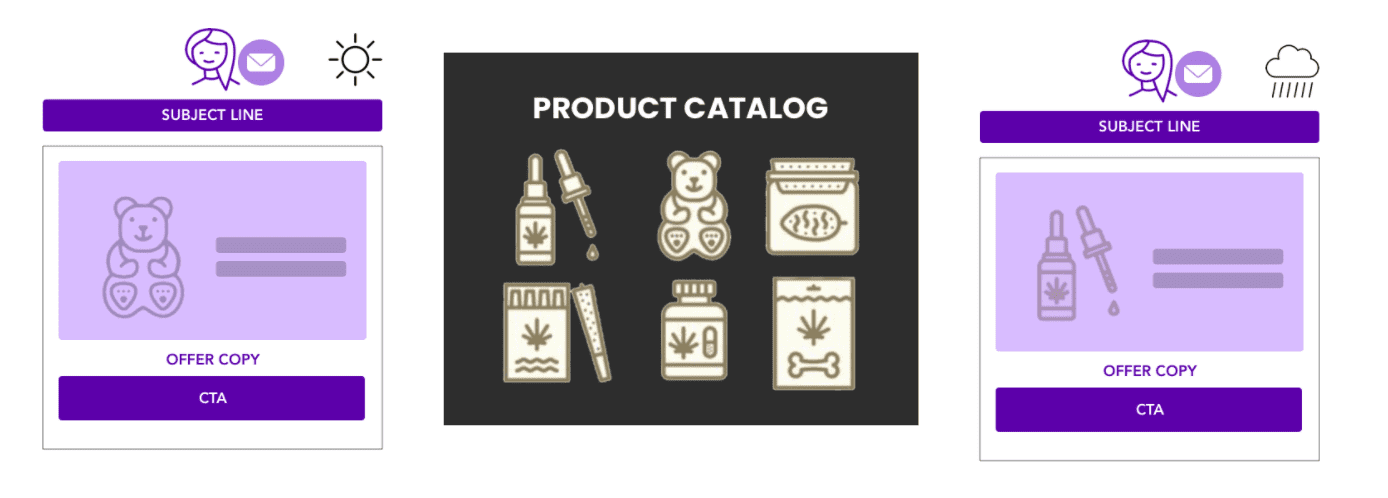
Weather can be added as context to provide customers with an even more personalized experience.
Step 4: Design Dynamic Templates
After the groundwork is completed in Steps 1 through 3, designing templates with dynamic data via handlebars and conditional sections via handlebar logic is only limited by the strategies a marketer can conjure.Â
The scale of personalization via dynamic templates RFM enables inside Iterable is astounding. In our experience with customers, a single, well-designed template can serve all eight personas simultaneously with persona-driven copy—all while presenting personalized products.
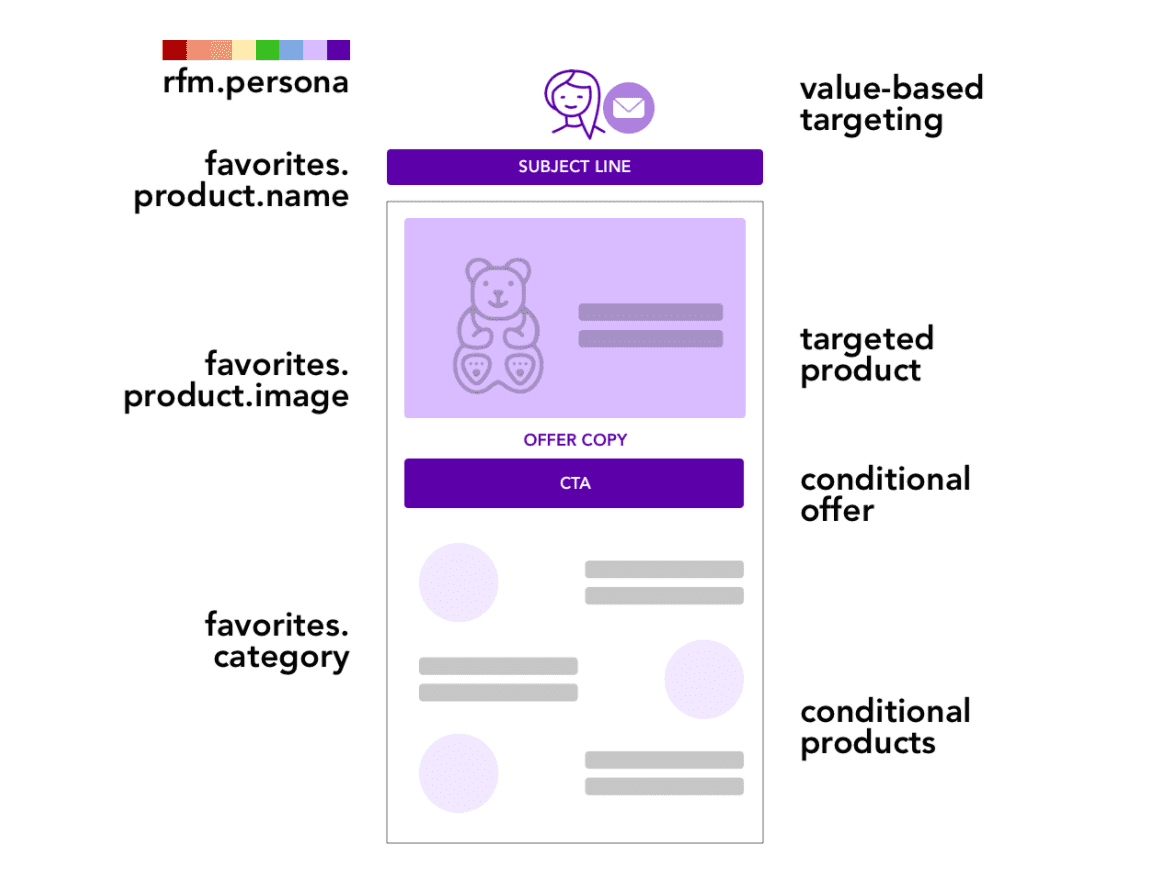
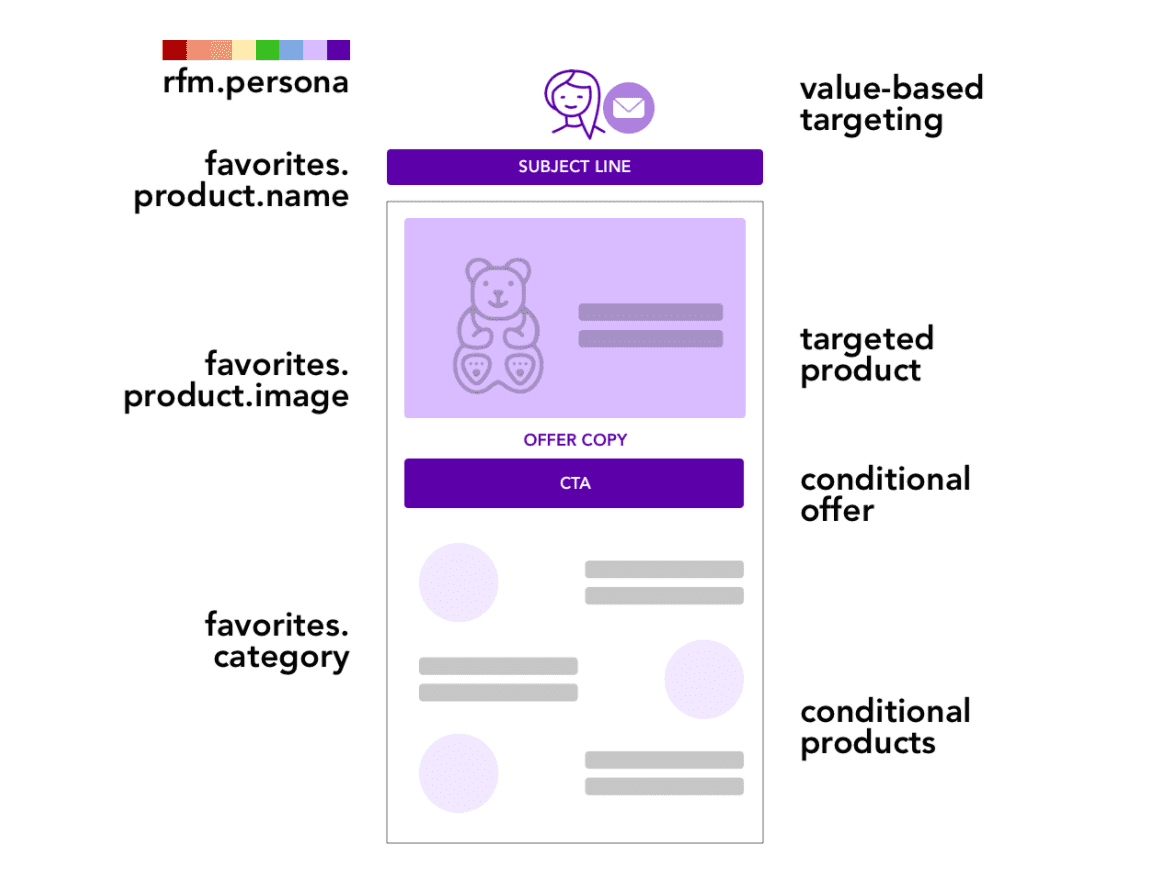
Dynamic templates can be designed to serve each persona.
Step 5: Leverage Semantic Data Models
The last step is the most critical because it provides the data feedback loop necessary to drive decisions. We leverage semantic data models (SDM) in the UTMs of our Iterable templates.Â
A semantic data model is a highly structured, intentionally-designed naming convention. The power in semantic data models is in the downstream application, as they can be parsed into multiple columns in Excel and then pivoted to arrive at data insights.Â
Segmentation analysis using SDM enables you to make smarter decisions by visualizing changes in promotional fatigue, offer elasticity, revenue contribution, and more.
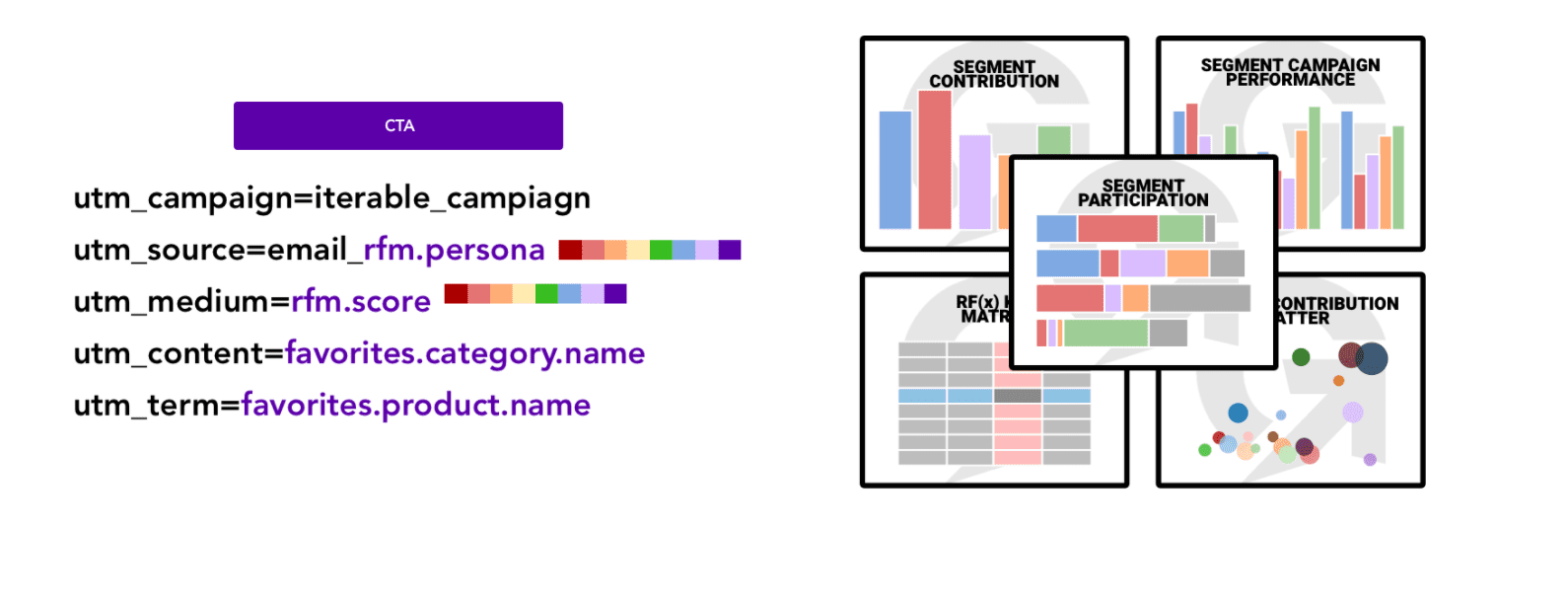
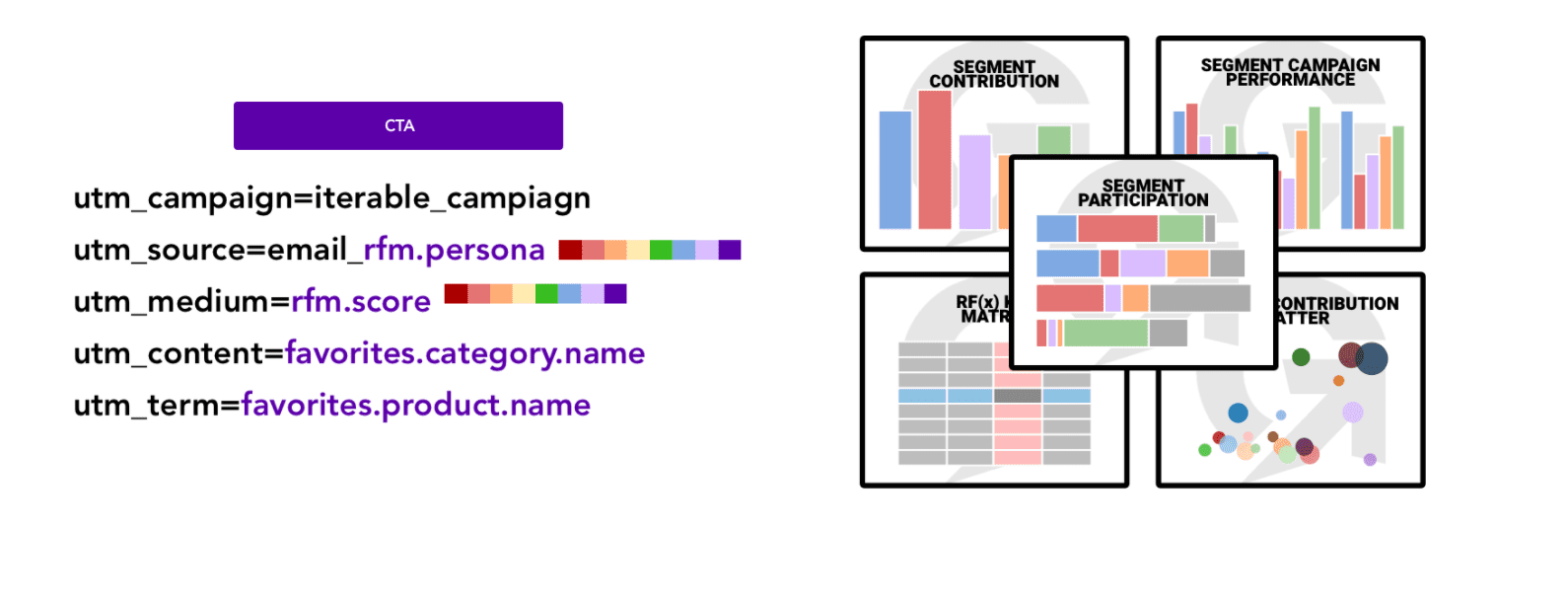
Semantic data models help you visualize changes in customer behavior.
With all five steps in place, you are able to continuously monitor the different segments of your RFM framework and act quickly to stimulate longer, more personalized relationships with your customers.
Segmentation at Its Finest
As marketers, we’re always on the hunt for more insight into our customers’ behavior. What drives their purchase decisions? What makes them tick?
RFM provides that granular, individualized insight through value-based segmentation. It’s easy to implement and easily integrated into your cross-channel marketing strategies. Once implemented, RFM can be utilized as quickly as your next promotional campaign.
When looking at your customer lifetime value and ways to improve it, RFM is that secret weapon you can’t afford to ignore.Â
Customer lifetime value is key to a memorable customer experience. Learn more about LTV at Activate Live on April 7!